by Robert A. Muenchen, updated March 31, 2025
Introduction
JASP is a free and open-source statistics package that targets beginners looking to point-and-click their way through analyses. This article is one of a series of reviews that aim to help non-programmers choose the Graphical User Interface (GUI) for R which best meets their needs. Most of these reviews also include cursory descriptions of the programming support that each GUI offers.
I have joined the BlueSky Statistics development team and have written the BlueSky User Guide (online here), but you can trust this series of reviews, as I describe here. All my comments below are easily verifiable. There is no perfect user interface for everyone; each GUI for R has features that appeal to different people.
JASP stands for Jeffreys’ Amazing Statistics Program, a nod to the Bayesian statistician Sir Harold Jeffreys. It is available for Windows, Mac, and Linux, and there is even a cloud version. One of JASP’s key features is its emphasis on Bayesian analysis. Most statistics software emphasizes a more traditional frequentist approach; JASP offers both.
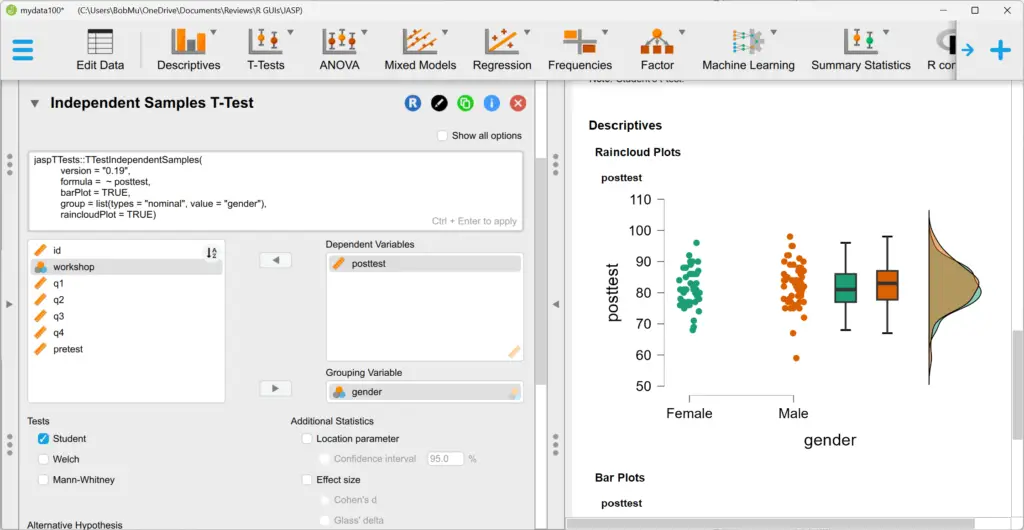
Terminology
There are various definitions of user interface types, so here’s how I’ll be using the following terms. Reviewing R GUIs keeps me quite busy, so I don’t have time also to review all the IDEs, though my favorite is RStudio.
GUI = Graphical User Interface using menus and dialog boxes to avoid having to type programming code. I do not include any assistance for programming in this definition. So, GUI users are people who prefer using a GUI to perform their analyses. They don’t have the time or inclination to become good programmers.
IDE = Integrated Development Environment, which helps programmers write code. I do not include point-and-click style menus and dialog boxes when using this term. IDE users are people who prefer to write R code to perform their analyses.
Reproducibility = The ability to record and re-run every detail of an analysis, preferably integrated with the text that describes those results. Most R GUIs offer code reproducibility, the ability to record the R code that you can use to reproduce the workflow. However, GUI users often don’t understand the code and have trouble re-purposing it for similar analyses. GUI reproducibility is the ability to save the workflow in GUI form. That is usually the dialog boxes and their settings that created the original complete analysis.
Installation
The various user interfaces available for R differ greatly in how they’re installed. Some, such as BlueSky Statistics, jamovi, and RKWard, install in a single step. Others install in multiple steps, such as R Commander (two steps) and Deducer (up to seven steps). Advanced computer users often don’t appreciate how lost beginners can become while attempting even a simple installation. The HelpDesks at most universities are flooded with such calls at the beginning of each semester!
JASP’s single-step installation is extremely easy and includes its own copy of R. So, if you already have a copy of R installed, you’ll have two after installing JASP. That’s a good idea, though, as it guarantees compatibility with the version of R that it uses, plus a standard R installation by itself is harder than JASP’s.
Plug-in Modules
When choosing a GUI, one of the most fundamental questions is: what can it do for you? What the initial software installation of each GUI gets you is covered in the Graphics, Analysis, and Modeling sections of this series of articles. Regardless of what comes built-in, it’s good to know how active the development community is. They contribute “plug-ins” that add new menus and dialog boxes to the GUI. This level of activity ranges from very low (Deducer, RKWard) to very high (BlueSky, R Commander).
For JASP, plug-ins are called “modules,” and they are found by clicking the “+” sign at the top of its main screen. That brings up a checklist of modules. Checking the box for one downloads and installs it. It is a very easy approach to use.
Currently, there are fourteen add-on modules for JASP that add 191 dialogs to it as of August 23, 2024:
- AUDIT
- BAIN
- BSTS
- Circular
- Cochrane Meta-Analysis
- Distributions
- Quality Control & DOE
- Equivalence T-Tests
- JAGS
- Learn Bayes
- Learn Stats
- Machine Learning
- Meta Analysis
- Network Analysis
- Power
- Predictive Analytics
- Prophet
- Process
- Quality Control & DOE
- Reliability
- Robust t-tests
- SEM
- Summary Stats
- Survival
- Time Series
- Visual Modeling
Startup
Some user interfaces for R, such as BlueSky, jamovi, and Rkward, start by double-clicking on a single icon, which is great for people who prefer not to write code. Others, such as R commander and Deducer, have you start R, load a package from your library, and then call a function to activate the GUI finally. That’s more appropriate for people looking to learn R, as those are among the first tasks they’ll have to learn.
You start JASP directly by double-clicking its icon from your desktop or choosing it from your Start Menu (i.e., not from within R itself). It interacts with R in the background; you never need to know that R is running.
Data Editor
A data editor is a fundamental feature in data analysis software. It puts you in touch with your data and lets you get a feel for it, if only in a rough way. A data editor is such a simple concept that you might think there would be hardly any differences in how they work in different GUIs. While there are technical differences, to a beginner, the differences in simplicity matter the most. Some GUIs, including BlueSky and jamovi, let you create only what R calls a data frame. They use more common terminology and call it a data set: you create one, you save one, later you open one, then you use one. Others, such as RKWard trade this simplicity for the full R language perspective: a data set is stored in a workspace. So the process goes: you create any number of data sets, you save them in a workspace, you open a workspace, and choose a dataset from within it.
JASP’s data editor offers the usual features of data entry, adding columns, and rows. It has a unique feature called Synchronisation, which sends your changes back out into an external non-JASP file (e.g., a text file) if one exists. This is important for two reasons. First, programs that store data along with output, notes, etc. mean you end up with copies of any external data files that need synchronization if you edit either copy. Synchronization also allows you to apply a complete set of analyses to a new dataset (with the same variable names) by simply switching the synchronization file. That’s complete GUI-based reproducibility.
The JASP data editor allows you to edit the metadata, such as variable names, scales, and values (factor levels). It uses statistical terminology, such as nominal, rather than R terminology, such as factor.
JASP cannot handle date/time variables other than by reading them as characters and converting them to factors. Once JASP decides a character or date/time variable is a factor, it cannot be changed.
Double-clicking on the name of a factor will open a small window on the top of the data viewer where you can overwrite the existing variable names and labels, as shown in Figure 2.
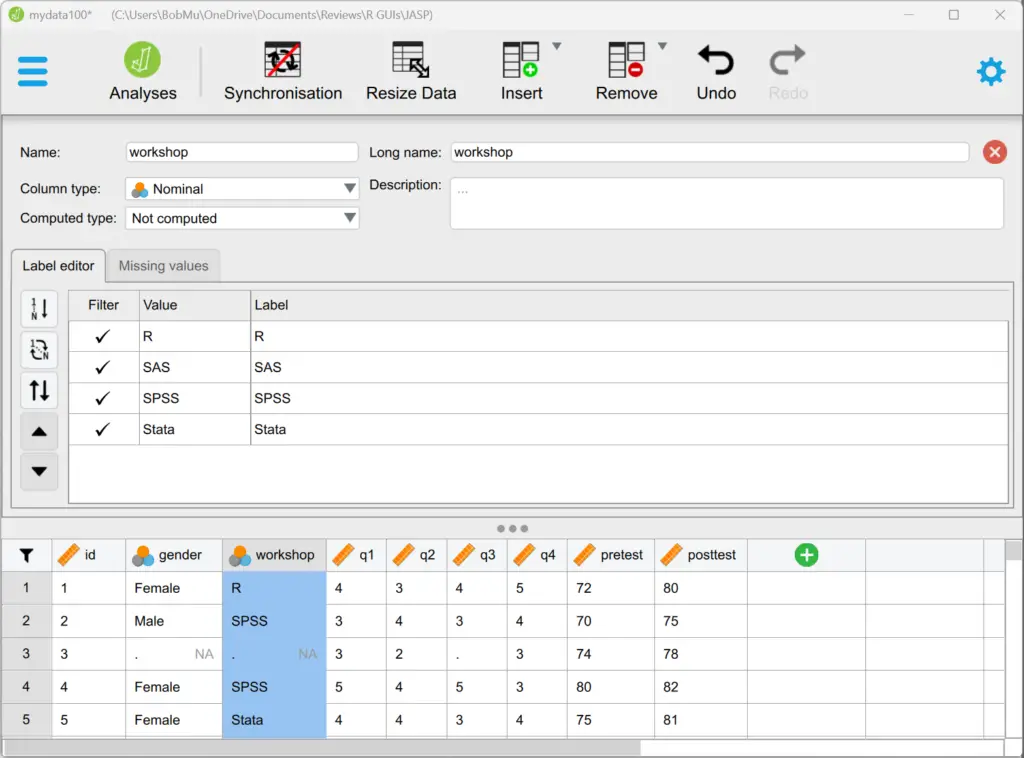
metadata window for that variable. Clicking the green Analysis icon in the upper left corner would hide the editor and display the dialogs and output shown in Figure 1.
If you have a large computer screen, you can see the data editor, dialogs, and output all at once, as shown in Figure 3. With this view, clicking the Edit Data icon on the upper left makes the editor cover the other two windows to give you the maximum view of your data.
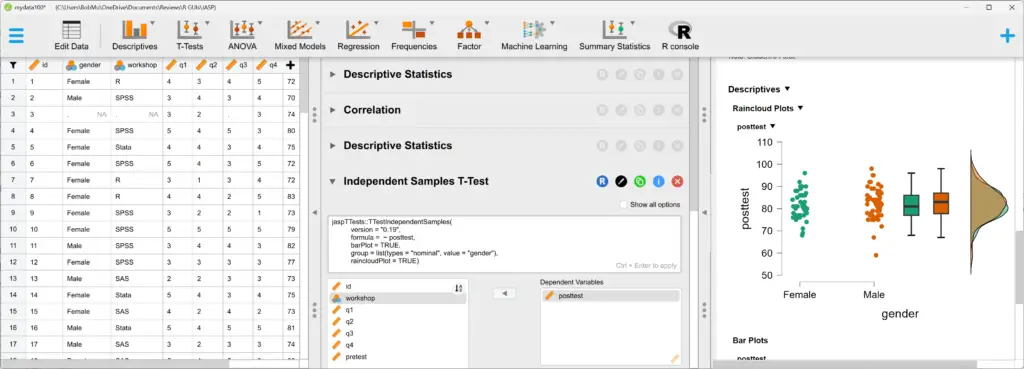
Data Import
The ability to import data from various formats is extremely important; you can’t analyze what you can’t access. Most GUIs evaluated in this series can open a wide range of file types and even pull data from relational databases. JASP can read from a wide range of data sources. Ironically, although it is based on R, JASP cannot read R data files!
- Delimited (.txt)
- Delimited (.csv)
- Delimited (.tsv)
- Excel (.xls)
- Excel .xlsx)
- SAS (.sas7bdat)
- SAS (.sas7bcat)
- SAS Export (.xpt)
- SPSS (.sav)
- SPSS (.zsav)
- SPSS Portable (.por)
- Stata (.dta)
- Open Document Spreadsheet (.ods)
- Open Science Framework
- Cochrane Library (for metanalysis)
JASP can also read the following databases.
- IBM DB2
- MySQL
- ODBC
- Oracle
- PostgreSQL
- SQLite version 3 or above
Data Export
The ability to export data to a wide range of file types helps when you need multiple tools to complete a task. Research is commonly a team effort, and in my experience, it’s rare to have all team members prefer to use the same tools. For these reasons, GUIs such as BlueSky, Deducer, and jamovi offer many export formats. Others, such as R Commander and RKward can create only delimited text files.
JASP doesn’t save just a dataset; instead, it saves the combination of a dataset plus its associated analyses. To save just the dataset, you go to the “File” tab and choose “Export Data.” The only export format is a comma-separated value file (.csv).
Data Management
It’s often said that 80% of data analysis time is spent preparing the data. Variables need to be computed, transformed, scaled, recoded, or binned; strings and dates need to be manipulated; missing values need to be handled; datasets need to be sorted, stacked, merged, aggregated, transposed, or reshaped (e.g., from “wide” format to “long” and back).
An essential aspect of data management is transforming many variables at once. For example, social scientists need to recode many survey items, biologists need to take the logarithms of many variables. Doing these types of tasks one variable at a time is tedious.
Some GUIs, such as BlueSky and R Commander, can handle nearly all of these tasks. Others, such as RKWard handle only a few of these functions.
JASP’s data management capabilities are minimal. It has a simple calculator that works by dragging and dropping variable names and math or statistical operators. Alternatively, you can type formulas using R code. Using this approach, you can only modify one variable at a time, making day-to-day analysis tedious. It’s also unable to apply functions across rows (jamovi handles this via a set of row-specific functions).
You can filter cases to work on a subset of your data. However, JASP can’t sort, stack, merge, aggregate, transpose, or reshape datasets. The lack of combining datasets may result from the fact that JASP can only open one dataset in a given session.
Menus & Dialog Boxes
The goal of pointing and clicking your way through an analysis is to save time by recognizing menu settings rather than performing the more difficult task of recalling programming commands. Some GUIs, such as BlueSky and jamovi, make this easy by sticking to menu standards and using simpler dialog boxes; others, such as RKWard, use non-standard menus that are unique to it and hence require more learning.
JASP’s interface uses a combination of standard menus and dialogs. The only non-standard aspects are how to juggle the data, dialog, and output areas if you have a small computer screen. You accomplish this with a combination of left/right arrow keys to shunt them about, and the Analyses/Data icons that flip between the data editor and the other two windows.
Clicking on any icon on the toolbar causes a standard dialog box to pop out the right side of the data viewer (e.g., Figure 3, center). You select variables to place into their various roles. This is accomplished by either dragging the variable names or selecting them and clicking an arrow next to the particular role box. As soon as you fill in enough options to perform an analysis, its output appears instantly in the output window to the right. After that, every option chosen adds to the output immediately; every option turned off removes output. The dialog box does have an “OK” button, but rather than cause the analysis to run, it merely hides the dialog box, making room for more space for the data viewer and output. Clicking on the output itself causes the associated dialog to reappear, allowing you to make changes. Those changes will replace the output from which you recalled the dialog. Choosing a dialog from the main menu places the output at the bottom of all previous output.
While nearly all GUIs keep your dialog box settings during your session, JASP keeps those settings in its main file. This allows you to return to a given analysis at a future date and try some model variations. You only need to click on the output of any analysis to have the dialog box appear to the right of it, complete with all settings intact.
Output is saved by using the standard “File> Save” selection.
Documentation & Training
The JASP Materials web page provides links to a helpful array of information to get you started. The How to Use JASP web page offers a cornucopia of training materials, including blogs, GIFs, and videos. The free book Statistical Analysis in JASP: A Guide for Students covers the software’s basics and includes a basic introduction to statistical analysis.
Help
R GUIs provide simple task-by-task dialog boxes, which generate much more complex code. So for a particular task, you might want to get help on 1) the dialog box’s settings, 2) the custom functions it uses (if any), and 3) the R functions that the custom functions use. Nearly all R GUIs provide all three levels of help when needed. The notable exception is the R Commander, which lacks help on the dialog boxes themselves.
JASP’s help files are activated by choosing the “i” icon for information in the upper right corner of any dialog box. This opens a new window containing the help. The help files are very well done, explaining what each choice means, its assumptions, and even journal citations. While there is no reference to the R functions used, nor any link to their help files, the overall set of R packages JASP uses is listed here.
Graphics
The various GUIs available for R handle graphics in several ways. Some, such as RKWard, focus on R’s built-in graphics. Others, such as BlueSky, focus on R’s popular ggplot graphics. GUIs also differ greatly in how they control the style of the graphs they generate. Ideally, you could set the style once, and then all graphs would follow it.
There is no “Graphics” menu in JASP; all the plots are created from within the analysis dialogs. It took me quite a while to get used to their locations. For example, boxplots are found in “T-Tests> Independent Samples T-Test> Raincloud Plots.” That yields the plot shown in Figure 1. Note that while it does contain a boxplot, it also contains strip and density plots, which I was not trying to get. I found no way to get just a boxplot.
Scatterplots are under “Regression> Correlation> Plots.” That created the plot shown in Figure 4. JASP is clearly ready for a scatterplot matrix, but with only two variables, the plot looks odd. Clicking the “display pairwise” option removed the empty space, resulting in a single scatterplot.
The plots JASP creates are well done, with a white background and axes that don’t touch at the corners. You can also set the graphics style you prefer; the following plots will adopt that style.
JASP uses its own custom functions for graphics, rather than R’s default graphics package, ggplot2, or lattice. People hoping to learn the R language might be more interested in seeing those alternatives.
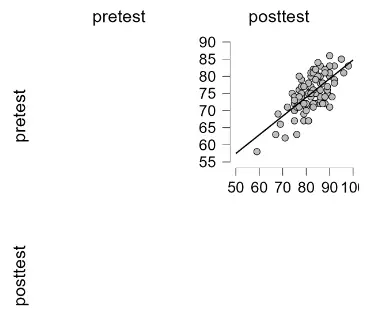
The plug-in called Visual Modeling adds the ability to do a single scatterplot and strip plots with error bars. You can also easily add panel variables (a.k.a. facets) to create small multiple plots with common axes, e.g., Figure 5.
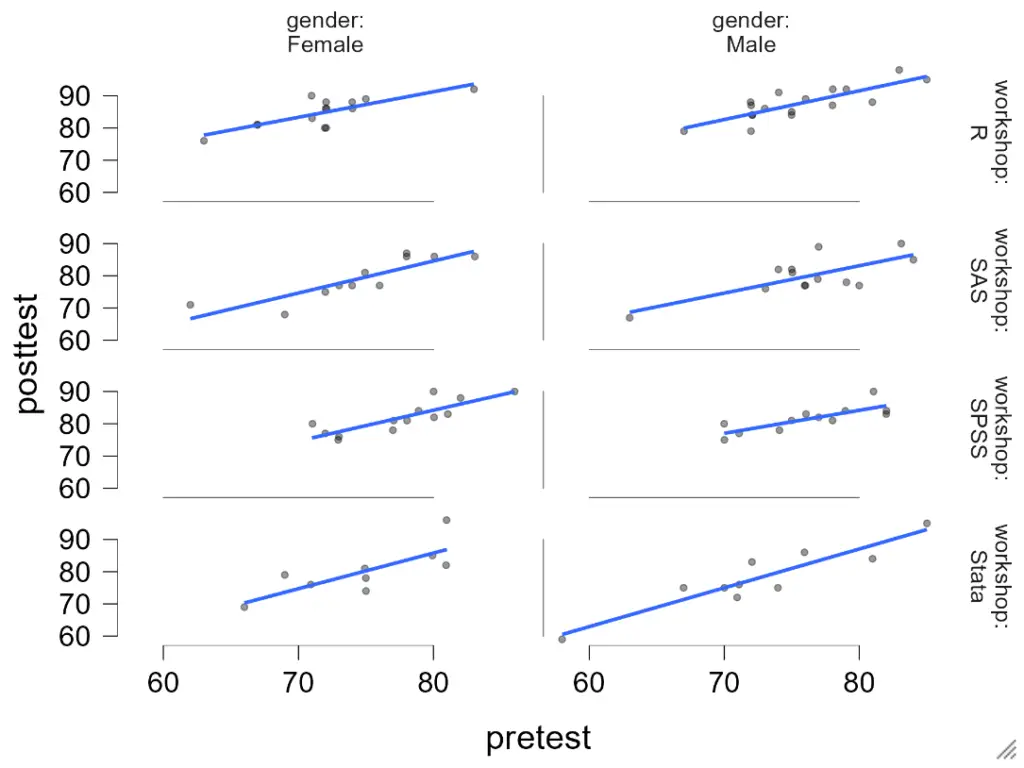
Here is the selection of plots JASP can create.
- Histogram
- Density
- Dot Plots
- Box Plots
- Bar Plots (means)
- Confidence intervals
- Density
- Interval Plots (mean point with confidence intervals)
- Likert Plots
- Machine Learning: Data Split Plot
- Machine Learning: Out-of-bag Improvement
- Machine Learning: ROC Curves Plot
- Machine Learning: Andrews Curves
- Machine Learning: Classification Accuracy
- Machine Learning: Deviance
- Machine Learning: Relative Influence
- Machine Learning: Decision Boundary Matrix Plot
- Pareto
- Pie Chart
- Q-Q Plot
- Raincloud Plot
- Scatterplot matrix
- Stem and Leaf Plot
- Strip Plots
- Violin Plots
- Flexplot> Scatterplot (single, in Flexplot)
- Flexplot> Interaction plots
- Flexplot> Strip plot with means with confidence intervals
Modeling
The way statistical models (which R stores in “model objects”) are created and used is an area in which R GUIs differ the most. Some, like RKWard, use a one-step approach to modeling. That approach tries to do everything you need in a single dialog box. This is perfect for beginners who appreciate being reminded of the various assumption tests and evaluation steps to take. However, for an R programmer, that approach seems confining since R can do a lot of different tasks with model objects. However, neither SAS nor SPSS could save models for their first 35 years of existence, so each approach has its merits. For simple models, like linear regression, standard compute statements can enter models to make predictions. Entering them manually is not much effort, and it saves you from learning what a model object is. However, some of the most powerful model types are essentially impossible to enter by hand, such as neural networks, random forests, and gradient-boosting machines.
Other GUIs, such as BlueSky and R Commander, do modeling using a two-step process. First, you generate and save a model, then use it for scoring new datasets, calculating model-level measures of fit or observation-level scores of influence, diagnostic plotting, testing differences between models, and so on.
JASP usually follows the one-step approach. It does not save models in its base package, so you must use compute statements to enter a model and apply it to a new data set (or a hold-out sample) to see how effectively the model generalizes. Instead, it tries to anticipate your needs, providing things like normal probability plots for residuals. This is great for intro statistics courses but lacks the flexibility that more advanced researchers might prefer. JASP has an add-on module for machine learning that offers a way to save models. Perhaps JASP’s internal modeling functions will eventually work similarly. While you can use JASP’s reproducibility to apply your modeling steps to a new dataset, it would be recalculating the model in that case, not applying an existing one.
Another way in which R GUIs differ is the model formula builder. Some, like jamovi and RKWard, offer only the most popular model types, providing interactions and allowing you to force the y-intercept through zero. Others, such as R Commander and BlueSky, offer maximum power by including buttons to control nested factors, polynomials, splines, etc.
JASP’s base modeling keeps model formulas simple. It adds the variables you select and all their possible interactions. That can generate a warning that you don’t have enough degrees of freedom, allowing you to remove terms then. Since JASP’s model builder does not offer advanced features like nested effects and splines, so those models cannot be done. The model it builds is not displayed as a standard R formula, which you could modify manually.
Analysis Methods
All of the R GUIs offer a decent set of statistical analysis methods. Some also offer machine learning methods. As you can see from the table below, JASP offers both. Included in many of these are Bayesian measures, such as credible intervals. See the Plug-in Modules section above for more analysis types.
Generated R Code
One of the aspects that most differentiates the various GUIs for R is the code they generate. If you decide to save code, what type is best for you? The base R code as provided by the R Commander, which can teach you “classic” R? The tidyverse code generated by BlueSky Statistics? The completely transparent (and complex) traditional code provided by RKWard, which might be the best for budding R power users?
JASP uses custom code for all its work. It’s very concise code that is easy to read, and it saves you from learning many functions from various R packages. However, if you hope to learn R eventually, you might prefer to learn a more standard set of functions.
Support for Programmers
Some of the GUIs reviewed in this series of articles include extensive support for programmers. For example, RKWard offers much of the power of Integrated Development Environments (IDEs) such as RStudio or Eclipse StatET. Others, such as jamovi or the R Commander, offer just a text editor with some syntax checking and code completion suggestions.
JASP’s R console is not really an editor. It lets you enter a command at a time, and the commands vanish once executed.
Reproducibility & Sharing
One of the biggest challenges that GUI users face is being able to reproduce their work. Reproducibility is useful for re-running everything on the same dataset if you find a data entry error. It’s also useful for applying your work to new datasets so long as they use the same variable names (or the software can handle name changes). Some scientific journals ask researchers to submit their files (usually code and data) along with their written reports so that others can check their work.
As important a topic as it is, reproducibility is a problem for GUI users, a problem that has only recently been solved by some software developers. Most GUIs (e.g. the R Commander, Rattle) save only code, but since GUI users don’t write the code, they also can’t read it or change it! Others, such as BlueSky, jamovi, and RKWard, save the dialog box entries and allow GUI users reproducibility in the preferred form.
JASP records nearly all the steps in an analysis and any notes you add providing reproducibility. The only thing it doesn’t save is manual data edits for the case in which your data come from a database to which you don’t have write access. Only BlueSky (and Stata) off that level of reproducibility). However, if you change a data value, all the analyses that used that variable are recalculated instantly. You can also use its synchronization feature to open a new data file and rerun all analyses on that new dataset.
If you wish to share your work with a colleague so they can execute it, perhaps with their modifications, they must be JASP users. There is no way to export an R program file for them to use. You only need to send them your JASP file; it contains the data and the steps you used to analyze it. For simply reading JASP output, it has a viewer in the Open Science Framework (https://osf.io/dashboard). You can store your .jasp file there so other users can see the output, including annotations.
Package Management
A topic related to reproducibility is package management. One of the major advantages of the R language is that it’s very easy to extend its capabilities through add-on packages. However, updates in these packages may break a previously functioning analysis. Years from now, you may need to run a variation of an analysis, which would require you to find the version of R you used, plus the packages you used at the time. As a GUI user, you’d also need to find the version of the GUI that was compatible with that version of R.
Some GUIs, such as the R Commander and Deducer, depend on you to find and install R. For them, the problem is left for you to solve. Others, such as BlueSky, distribute their own version of R, all R packages, and all its add-on modules. This requires a bigger installation file, but it makes dealing with long-term stability as simple as finding the version you used when you last performed a particular analysis. Of course, this depends on all major versions being around for the long term. Still, for open-source software, multiple archives are usually available to store software, even if the original project is defunct.
JASP is firmly in the safer camp. It provides nearly everything you need in a single download. This includes the JASP interface, R itself, and all R packages it uses.
Output & Report Writing
Ideally, output should be clearly labeled, well organized, and of publication quality. It might also delve into word processing through R Markdown, knitr, or Sweave documents. Currently, none of the GUIs covered in this series of reviews meet all of these requirements. See the separate reviews to see how each package is doing on this topic.
The labels for each of JASP’s analyses are provided by a single main title, which is editable. Clicking on a pen icon lets you edit the title. Clicking on a title in the output brings up a menu offering to add a note to display in the output.
JASP has a table of contents (TOC) feature that is extremely well done. Each step in your analysis appears in the TOC automatically. Clicking on its entry drops the dialog down into view, where you can change and rerun it. The new output for a recalled dialog replaces the original. Dialogs chosen from the main menu appear at the bottom of the output. You can change the order of the steps by simply dragging their titles around. This is the most well-done TOC of any of the R GUIs I have reviewed. I hope the others follow JASP’s lead on this.
JASP’s output quality is very high, with nice fonts and true rich text tables (Table 2). Tabular output is displayed in the popular style of the American Psychological Association. That means you can right-click on any table and choose “Copy,” and the formatting is retained. That helps speed your work as R output defaults to mono-spaced fonts that require additional steps to get into publication form (e.g. using functions from packages such as xtable or texreg). You can also export an entire set of analyses to HTML and then open the nicely formatted tables in Word. JASP lets you enter long descriptive variable names, which appear in the output. However, it does not offer the classic variable labels feature, which allows one to choose between short names, long labels, or both in the output. Of the software reviewed in this series, only RKWard and BlueSky Pro offer that.
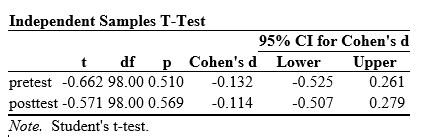
LaTeX users can click the downward arrow at the top of each output table and choose ” Copy LaTeX” to recreate the table in that text formatting language.
Group-By Analyses
Repeating an analysis on different groups of observations is a core task in data science. Software needs to provide the ability to select a subset of one group to analyze and then another subset to compare it to. All the R GUIs reviewed in this series can do this task.
JASP offers three ways to select subsets. The easiest is to double-click on a variable’s name in the data editor. The variable descriptive information and a filter column shows all the variable’s values. Unchecking any value removes such observations from the data. A more flexible approach is to use the funnel icon in the data editor’s upper left corner. Clicking it opens a window that allows you to use icons of logical symbols to build your selection logic, such as “gender = Female.” That saves beginners from knowing that R uses “==” for logical equivalence. Nor do beginners need to know to enclose strings in quotes. Finally, you can type a filter using R code.
When a filter is set, the data viewer grays out the excluded data lines to give you a visual cue. An eye icon lets you hide the suppressed observations if you like. Regardless of how you create a filter, it generates a subset that immediately changes all the previous results you ran. That surprised me as I’m used to having one report showing, for example, a set of steps for males and then again for females. In JASP, you would have to choose one subset, save the report, choose the other subset, save that report, and then use another tool to combine the reports.
Software also needs the ability to automate such selections so that you might generate dozens of analyses, one group at a time. While this has been available in commercial GUIs for decades (e.g. SPSS “split-file,” SAS “by” statement), BlueSky is the only R GUI reviewed here that includes this feature. The closest JASP gets to this topic is offering a “split” variable selection box in its Descriptives procedure.
Output Management
Early in the development of statistical software, developers tried to guess what output would be important to save to a new dataset (e.g. predicted values, factor scores), and the ability to save such output was built into the analysis procedures themselves. However, researchers were far more creative than the developers anticipated. To better meet their needs, output management systems were created and tacked on to existing tools (e.g., SAS’ Output Delivery System, SPSS’ Output Management System). One of R’s greatest strengths is that every bit of output can be readily used as input. However, with the simplification that GUIs provide, that’s a challenge.
Output data can be observation-level, such as predicted values for each observation or case. When group-by analyses are run, the output data can also be observation-level, but now the (e.g.) predicted values would be created by individual models for each group, rather than one model based on the entire original data set (perhaps with group included by a set of indicator variables).
You can also use group-by analyses to create model-level data sets, such as one R-squared value for each group’s model. You can also create parameter-level data sets, such as the p-value for each regression parameter for each group’s model. (Saving and using single models is covered under “Modeling” above.)
For example, in our organization, we have 250 departments and want to see if any have a gender bias on salary. We write all 250 regression models to a dataset and then search to find those whose gender parameter is significant (hoping to find none, of course!)
BlueSky is the only R GUI reviewed here that does all three levels of output management. JASP not only lacks these three levels of output management, it even lacks many of the fundamental observation-level saving that SAS and SPSS offered in their first versions back in the early 1970s. It will save residuals from regression models, but not predicted values and factor or principal component scores, but that is all.
Developer Issues
While most of the R GUI projects encourage module development by volunteers, the JASP project hasn’t done so. However, this is planned for a future release.
Conclusion
JASP is easy to learn and use. The tables and graphs it produces follow the guidelines of the American Psychological Association, making them acceptable to many scientific journals without any additional formatting. Its table of contents makes it easy to restructure a report. Its developers have chosen their options carefully, so each analysis includes what a researcher wants to see. Its coverage of Bayesian methods is the most extensive I’ve seen in this series of software reviews. Only jamovi offers a similar set, since its developers copied the same code from JASP to jamovi. One of JASP’s best features is its ability to reuse a set of analyses on a new dataset via its data synchronization feature.
As nice as JASP is, it lacks important features, including a full R code editor, the ability to handle date/time variables, the ability to perform many fundamental data management tasks, the ability to save new variables such as predicted values, and the ability to create nested or spline models.
Acknowledgments
Thanks to Eric-Jan Wagenmakers, Bruno Boutin, and Thomas Langkamp for their help in understanding JASP’s finer points. Thanks also to Rachel Ladd, Ruben Ortiz, Christina Peterson, and Josh Price for their editorial suggestions.