By Robert A. Muenchen, updated 5/30/2023
Having completed several detailed reviews of Graphical User Interfaces (GUIs) for R, let’s compare them. It’s not too difficult to rank them based on the number of features they offer, so let’s start there. I’m basing the counts on the number of dialog boxes in each category of four categories:
- Ease of Use
- General Usability
- Graphics
- Analytics
This is trickier data to collect than you might think. Some software has fewer menu choices, depending instead on more detailed dialog boxes. Studying every menu and dialog box is very time-consuming, but that is what I’ve tried to do to keep this comparison trustworthy. I’m putting the details of each measure in the appendix so you can adjust the figures and create your own categories. If you decide to make your own graphs, I’d love to hear from you in the comments below.
Figure 1 shows how the various GUIs compare on the average rank of the four categories. R Commander is abbreviated Rcmdr, and R AnalyticFlow is abbreviated RAF. We see that BlueSky is in the lead, with R-Instat close behind. As my detailed reviews of those two point out, they are extremely different pieces of software! Rather than spend more time on this summary plot, let’s examine the four categories separately.
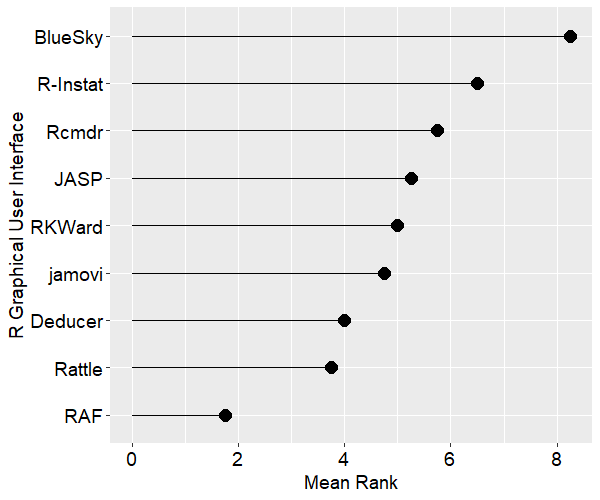
For the category of ease-of-use, I’ve defined it primarily by how well each GUI does what GUI users are looking for: avoiding code. They get one point each for being able to install, start, and use the GUI to its maximum effect, including publication-quality output, without knowing anything about the R language itself. Figure two shows the result. JASP comes out on top here, with jamovi and BlueSky right behind.
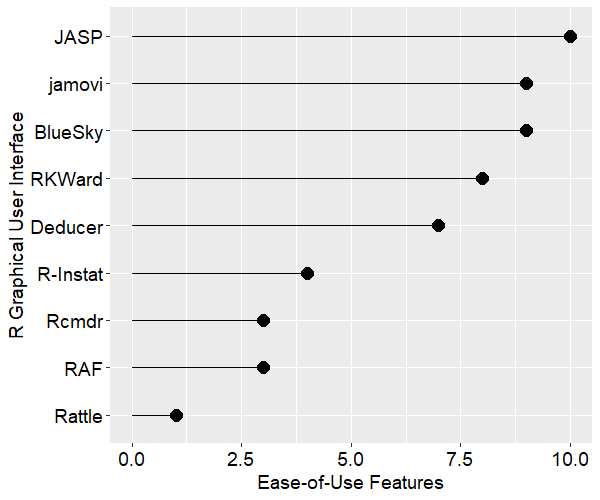
Figure 3 shows the general usability features each GUI offers. This category is dominated by data-wrangling capabilities, where data scientists and statisticians spend most of their time. This category also includes various types of data input and output. BlueSky and R-Instat come out on top not just due to their excellent selection of data-wrangling features but also due to their use of the rio package for importing and exporting files. The rio package combines the import/export capabilities of many other packages, and it is easy to use. I expect the other GUIs will eventually adopt it, raising their scores by around 40 points. JASP shows up at the bottom of this plot due to its philosophy of encouraging users to prepare the data elsewhere before importing it into JASP.
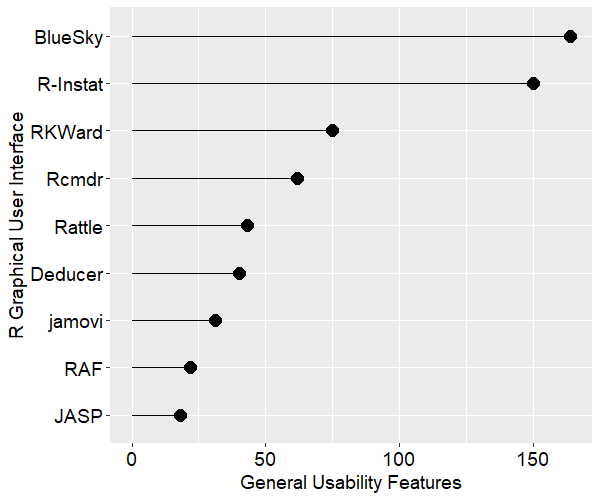
Figure 4 shows the number of graphics features offered by each GUI. R-Instat has a solid lead in this category. In fact, this underestimates R-Instat’s ability if you include its options to layer any “geom” on top of any graph. However, that requires knowing the geoms and how to use them. That’s knowledge of R code, of course.
When studying these graphs, it’s important to consider the difference between the relative and absolute performance. For example, relatively speaking, JASP and R Commander are not doing well here, but they do offer over 25 types of plots! That absolute figure might be fine for your needs.

Finally, we get to what is, for many people, the main reason for using this type of software: analytics. Figure 5 shows how the GUIs compare on the number of statistics, machine learning, and artificial intelligence methods. Here R Commander shows, well, a “commanding” lead! This GUI has been around the longest and so has had more time for people to contribute to its capabilities. If you read an earlier version of this article, R Commander was not as dominant. That was because I had not yet taken the time necessary to load and study every one of its 42 add-ons. That required a substantial effort, and these updated figures reflect a more complete view of its capabilities.
Again, it’s worth considering the absolute values on the x-axis. JASP and R-Instat are in the middle of the pack, but they both have over 200 methods. If that is sufficient for your needs, you can focus on the other categories.
Many important details are buried in these simple counts. For example, I enjoy using jamovi for statistical analyses, but it currently lacks machine learning and artificial intelligence. I like BlueSky too, but it doesn’t yet do any Bayesian statistics (jamovi and JASP do).
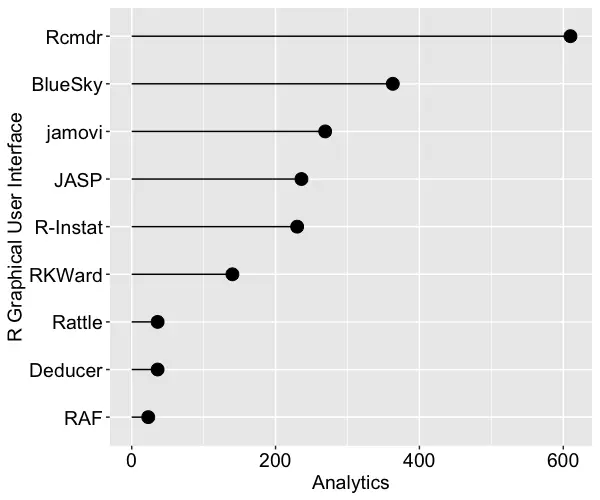
Overview of Each R GUI
The above plots help show us overall feature sets, but each package offers methods that the others lack. Let’s look at a brief overview of each. Remember that each of these has a detailed review that follows my standard template. I present them in alphabetical order.
BlueSky Statistics – This software was created by former SPSS employees, and it shares many of SPSS’ features, making it a good alternative. BlueSky is only a decade old, and it converted from commercial to open source mid-way through 2018. Its developers have been adding features at a rapid rate. Its adoption by the Mayo Clinic in 2022 raised its visibility substantially. When using BlueSky, it’s not initially apparent that R is involved at all. Unless you click the code button “</>” included in every dialog box, you’ll never see the R code. If you want to learn R code, seeing what BlueSky uses for each step can help. BlueSky saves the dialog settings for every step, providing GUI-based reproducibility. For R code, it uses the popular but controversial tidyverse style, while most of the other GUIs use base R functions or their own custom ones. BlueSky’s output is in publication-quality tables, which follow the popular style of the American Psychological Association. It’s stronger than most of the others in AI/ML and psychometrics. Its features for Six Sigma, quality control, and design of experiments make BlueSky a good alternative to Minitab and JMP. BlueSky is now available for Windows and Mac (previous versions were Windows-only). The user guide is online here. BlueSky is the only R GUI with a company supporting it, though that support is not free.
Deducer – This has a very nice-looking interface, and it’s probably the first R GUI to offer output in true APA-style word processing tables. Being able to just cut and paste a table into your word processor saves a lot of time, and it’s a feature that has been copied by several others. Deducer was released in 2008, and when I first saw it, I thought it would quickly gain developers. It got a few, but development seems to have halted. Deducer’s installation is quite complex, and it depends on the troublesome Java software. It also uses JGR, which never became as popular as the similar RStudio. The main developer, Ian Fellows, has moved on to another interesting GUI project called Vivid. I ran Deducer most recently in February 2022, and the output had many unknown characters in it, perhaps due to a lack of support for Unicode. Rest in peace, Deducer!
jamovi – The developers who form the core of the jamovi project used to be part of the JASP team. Even though they started a couple of years later, they’re ahead of JASP in several ways at the moment. Its developers decided that the R code it used should be visible and any R code should be executable, features that differentiate it from JASP (version .17 of JASP can display some R Code). jamovi has a highly interactive interface that immediately shows you the result of every selection in each dialog box (JASP does too). It also saves the settings in every dialog box and lets you re-use every step on a new dataset by saving a “template.” That’s extremely useful since GUI users often prefer to avoid learning R code. jamovi’s biggest weakness is its dearth of data management features, though there are plans to address that. The most recent version of jamovi borrowed most of the Bayesian analysis methods from JASP, making those two nearly tied as the leaders in that approach. jamovi can help you learn R code by showing what it does at each step, though it uses its own functions from the jmv package. While those functions are not standard R, they do combine the capability of many R functions in each one. If you deal with datasets over a million cases, note that jamovi is substantially slower than most of the other R GUIs.
JASP – The biggest advantage JASP offers is its emphasis on Bayesian analysis. If that’s your preference, this might be the one for you. Another strength is JASP’s Machine Learning module. Currently, JASP is very different from all the other GUIs reviewed here because it can’t show you all the R code it’s writing. Version 0.17 has recently started displaying some of its R code. JASP’s main weakness is its almost complete lack of data management features. It is commonly said that data scientists spend 80% of their time preparing data to analyze, almost none of which can be done in JASP. However, if you use spreadsheets or databases to “wrangle” your data, JASP may be the package for you. JASP 0.17 is unable to analyze large datasets (e.g., with a million cases, perhaps fewer) but is working to address the issue.
R AnalyticFlow – This is unique among R GUIs as it is the only one that lets you organize your analyses using flowchart-like workflow diagrams. That approach makes it easy to visualize what a complex analysis is doing and to rerun it. It writes very clean base R code and provides easy access to the powerful lattice graphics package. It also supports the ggplot2 graphics package, but only through its more limited quickplot function. R AnalyticFlow also lets you extend its capability making it easier for R power users to interact with non-programmers. However, it has some serious limitations. Its set of analytic and graphical methods is quite sparse. It also lacks the important advantage that most workflow-based tools have: the ability to re-use the workflow on a new dataset by changing only the data input nodes. Since each node requires the name of the dataset used, you must change it in each location.
Rattle – Just a few years ago, Rattle was easy to recommend for people who wanted to focus on learning machine learning methods. However, now several other R GUIs, such as JASP and BlueSky Statistics, offer more ML methods, many more statistical methods, they are as easy to use, and they install in a single step. Rattle’s incompatibility with recent versions of R and RStudio also gives me pause. I plan to track Rattle’s progress, and I hope to see it improve in future versions. However, until it does, I recommend trying some of the R GUI alternatives.
R Commander – This is the oldest GUI, having been around since at least 2005. There are an impressive 42 add-ons developed for it. It is currently one of only three R GUIs that saves R Markdown files (the others being BlueSky and RKWard), but it does not create word processing tables by default, as some of the others do. The R code it writes is classic, rarely using the newer tidyverse functions. It works as a partner to R; you install R separately, then use R to install and start R Commander. R Commander makes it easy to blend menu-based analysis with coding. If your goal is to learn to code using base R, this is an excellent choice. The software’s primary developer, John Fox, told me in January 2022 that he has no future development plans for R Commander. However, others can still extend its feature set by writing add-ons.
R-Instat – This offers one of the most extensive collections of data wrangling, graphics, and statistical analysis methods of any R GUI. At a basic level, its graphics dialogs are easy to use, and it offers powerful multi-layer support for people who are familiar with the ggplot2 package’s geom functions. However, to use it for even basic statistical tests, you must know and edit R code. Having to know that code is the main thing GUI users are trying to avoid! To use its full modeling capabilities, you need to know what R’s packages (e.g., MASS) do and how to use each one’s functions (e.g., rlm). For an experienced R programmer, recognizing a known “package::function” combination is much easier than recalling it without assistance. Such a user would find R-Instat’s GUI extremely helpful.
RKWard – This GUI blends a nice point-and-click interface with an integrated development environment (IDE) that is the most advanced of all the other GUIs reviewed here. It’s easy to install and start, and it saves all your dialog box settings, allowing you to rerun them. However, that’s done step-by-step, not all at once as jamovi’s templates allow. The code RKWard creates is classic R, with no tidyverse at all. RKWard is one of only three R GUIs that support R Markdown (the others: BlueSky and jamovi).
Conclusion
I hope this brief comparison will help you choose the R GUI that is right for you. Each offers unique features that can make life easier for non-programmers. Instructors of introductory classes in statistics or ML/AI should find these can enable their students to focus on the material rather than on learning the R language. If one catches your eye, don’t forget to read the full review of it here.
Acknowledgments
Writing this set of reviews has been a monumental undertaking. It would not have been possible without the assistance of Bruno Boutin, Anil Dabral, Ian Fellows, John Fox, Thomas Friedrichsmeier, Rachel Ladd, Jonathon Love, Ruben Ortiz, Danny Parsons, Christina Peterson, Josh Price, David Stern, Roger Stern, and Eric-Jan Wagenmakers, and Graham Williams.
Appendix: Guide to Scoring
The four categories are defined by the following. The yes/no items get scored 1 for yes, and 0 for no. The “how many” items consist of simple unweighted counts of the number of features, e.g., the number of file types a package can import without relying on R code. I used to plot the total number of features, but that is now dominated by the large values for analytics features, making that plot fairly redundant.
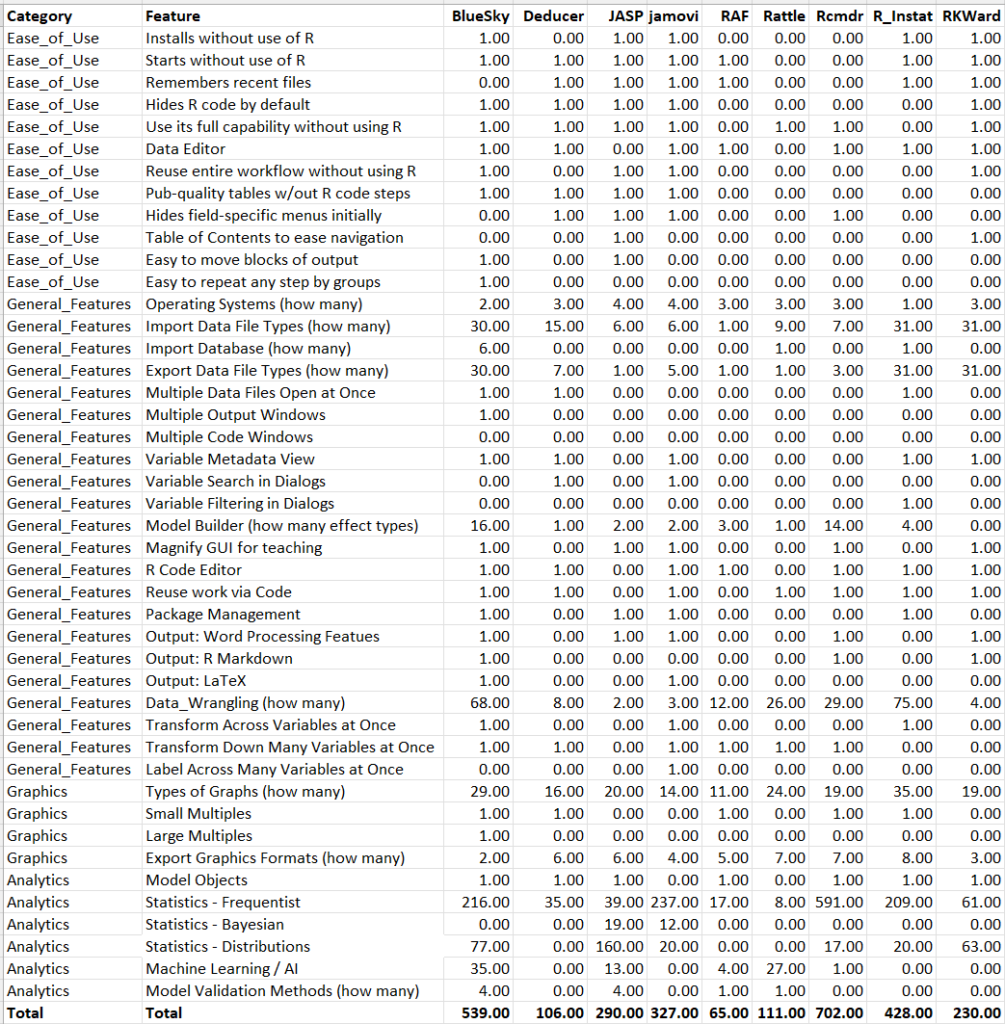
Why have you excluded RStudio? Very odd decision.
Hi Matty,
RStudio is a wonderful Integrated Developement Environment (IDE) that helps you control R via code, not graphical selections as the Graphical User Interfaces I review do. As I say in the post, “Each offers unique features that can make life easier for non-programmers. Instructors of introductory classes in statistics or ML/AI should find these can enable their students to focus on the material rather than on learning the R language.”
Cheers,
Bob